Artificial intelligence has landed in the world of stop loss and reinsurance, and this innovative set of tools has the potential to unlock new insights and data sets.
But how will AI providers filter out the noise and incorporate their models into this established $35 billion industry?
We’re going straight to the source with three key leaders in this space: Jason Oswald from Gradient, Ian Pietz from Prealize, and Ali Panjwani from Merit Medicine.
Together, we’ll explore the evolving landscape of predictive analytics in the stop loss and reinsurance sectors, including how artificial intelligence tools stand to redefine underwriting practices and their relationship with data access.
Ali will explain his “3-V” value proposition that AI can unlock in underwriting—visibility, variability, and value.
And all of our expert guests will lay out their visions for future broader adoptions of predictive analytics across the various carrier types.
Read the Transcript
Mehb Khoja
All right everybody, welcome back to Firm & Final. And for today’s discussion I have a group of individuals that work in the new and very innovative, even somewhat AI underwriting tool space. So I’m really excited to dive into this conversation today.
Now, before we get into introductions, I just I’m going to set up a scenario here for you. So this is what I think about when I think about you guys.
I think about a SIIA conference. I think about you guys being in that vendor space, the vendor set up space where people walk in and see all the different vendors that are at the conference. You guys all go back to the bar afterwards.
You have a drink together and you say to yourself, “What a bunch of idiots. These guys are in the stop loss and the reinsurance space. They don’t know anything about underwriting.”
And you guys have a very different approach. So we’re going to dive into that today. But just to get us started, we have with us today Jason Oswald, who is with Gradient, we have Ian Pietz who’s with Prealize, and we have Ali Panjwani who’s with Merit Medicine.
And just to get us started, can you guys give us a little bit of background on you, your company, and how long you’ve been in this space? Ali why don’t we start with you?
Ali Panjwani
Sure, Mehb. Thanks for having me. Ali Panjwani, Founder/CEO of Merit Medicine. I’ve been in the healthcare space for over a decade. Last ten years has been really focused on either supporting payers or negotiating with payers, primarily in the pharma and biotech side. So I was in market access for the majority of my career in the last few years, shifted over and really supporting the mission around affordability, predictability, better underwriting.
Mehb Khoja
Very cool. Ian, how about you?
Ian Pietz
I started out as a health actuary, so I started out working for a national payer and went through a number of different programs there. I worked in commercial pricing. I worked in level funded and PEO pricing. And really what I enjoyed most about that was the connection between insurance and protection for the individuals. And that’s why healthcare was so exciting to me from a financial perspective.
My background is in math. I transitioned out into one of the consulting shops and got into health underwriting predictive models. Those were things that I’d used on the payer side, and I joined Prealize here a little over a year ago as their product innovation VP, and really focused on taking the predictive models that Prealize had developed and finding applications for underwriting solutions.
Mehb Khoja
All right. Very cool. Jason, how about you?
Jason Oswald
Yeah, very similar to Ian, actually. Though the opposite path. So I came also came out of mathematics. I’ve been in health insurance now for about 15 years, a little over 15 years, and started in the consulting space with pooled arrangements and then true self-funded groups, then moved into the carrier side, handling both fully insured and self-funded type arrangements.
I’ve been at Gradient here for about three years. We’ve had a health practice for over five years now. The company itself started doing risk modeling with property and casualty. So we’ve been doing health, as I said, for about five years, both fully insured and now self-funded.
Mehb Khoja
Yeah. And so what do you guys call your space? Because sometimes people refer to it as AI underwriting. Sometimes people call it predictive underwriting. What do you guys refer to it as?
Jason Oswald
Yeah, I think my experience probably coming up to working at Gradient, I just refer to it as like a predictive underwriting. I kind of think broadly what we’re all doing is kind of the natural evolution of underwriting, which is we try to do the job better and we use new tools and data collection methods that are available kind of broadly and apply those.
So I still think it’s predictive underwriting. We just happen to be using this burgeoning tool set that’s out in the market right now with all the AI modeling.
Ian Pietz
I think we’re very similar, but I think we refer to ourselves as a predictive analytics company, and I think that’s part of what attracted me to this role. So we do the predictive underwriting. But Prealize was really founded about a decade ago focused on the care management side of the house. So we’ve spent a lot of time there on engagement, predicting conditions and what you could do about it, and then transitioned into the underwriting space over the last several years.
Mehb Khoja
Ali how about you?
Ali Panjwani
I think I’m pretty aligned with Ian’s description there. The way that I see it, our tools are here not to necessarily replace an actuary or an underwriter, but really just supercharge their abilities. There’s a lot of value in the experience that an underwriter or an actuary brings, and what we want to be able to do is process information at a greater volume, faster, and provide more accurate future insights.
What you do with it, how you price groups, is a function of how much risk you want to take as a company, but you know, we want to be a very valuable input into that process.
Mehb Khoja
Yeah. So, Ali, you founded a company around this. Ian and Jason, you guys are pretty new to your companies too in the past couple of years. So you must all think that there’s a really big opportunity. Dive into that for me. Why do you guys feel like there’s a big opportunity with predictive analytics, specifically in the stop loss and reinsurance community?
Ali Panjwani
I’d love to take this one first.
Mehb Khoja
Go for it.
Ali Panjwani
As cheeky as it sounds. I’ve got a bit of, an acronym for this. It’s the three V’s. So visibility, variability, and value.
So I’m going to kind of describe those in brief. So visibility I think is one of the most important needs in the space and why I got excited about it. The limited information that a lot of the carriers have when they’re first trying to underwrite a group and price the group is a huge gap.
So you know, only knowing a subset of information about a subset of members in every group, but having to underwrite the risk of the entire group, I think, is a really huge opportunity. So that’s visibility.
Variability is even when you know that somebody has a condition with a comorbidity who’s on maybe a disease modifying therapy. There’s a number of different paths that, that individual can take. And the cost outcomes are highly variable as well.
Depends on a number of things, access to care. And then the last one is value. So just purely there’s a firehose of claims data, labs data information out there and companies only have so many eyeballs to look at that stuff. And so technology just supercharges your ability to look at every detail and make sense of it.
Mehb Khoja
Ian, how about you?
Ian Pietz
Yeah I’ll jump in next, yeah I love what you said there, Ali, about the technology. To me that’s one of the things that makes the stop loss market interesting from a predictive perspective.
There’s been the use of these types of models in the fully insured space, I think for, you know, over a decade, but even longer with some of the kind of the statistical models. But access to the data needed for stop loss predictive modeling, the type of model architectures that are needed to predict, those very rare incident types of events, that’s, I think, relatively new, better and better data access is out there now.
The other thing is on the competitive side, so you’ve got more players in the space, more RFPs, low close rates. Talking about the operational efficiency aspect there, but finding ways to do your underwriting quickly and efficiently with low cost. I think that makes the market attractive as well.
Mehb Khoja
Jason, what are your thoughts?
Jason Oswald
Yeah. Your question was, you know, why do we move into the space? We think there’s an opportunity. I mean, frankly, I just found it interesting. There was, a lot of new things happening, new companies getting started. You know, other ones, like we have represented here on the call, they are just doing something very interesting.
And I think it’s obvious to me at least it was obvious it was adding value. I think Ian’s point’s a very good one about the availability of data. We’ve known that’s been there, but it’s also a huge volume. And so, we just saw a need. I just like the idea of actually being able to, to leverage and manage a lot of the information that was available and apply it to the space that I was in.
Mehb Khoja
Yeah. That’s interesting. I want to jump on something that Ian said. So you said that these tools have been around in the fully insured space for the past ten years, and in that space, if you’re the fully insured carrier and you have this group as a current group, you have all the data in the world, right? But when you’re in the stop loss space, like data stinks, right?
If done right, a group has no more than 2 to 4 stop loss claims a year. And if you’re getting data at 50% of that level, you might be seeing maybe five individuals that are at 50% of the threshold. And that’s it. Sometimes you get Joe Smith cancer diagnosis, $300,000 and that’s it. So tell me about your guys’ experience specifically in the stop loss space.
What has been your access to the data and how have you been able to help those customers of yours with your underwriting and predictive capabilities?
Ali Panjwani
It’s such an important question. So having a subset of those members and some visibility into those members is clearly not enough. And kind of the outcomes show it. From our perspective, it’s extremely important to get visibility into almost all members. So all members, if possible, but if not at least a very high percentage of the members.
Secondary what’s important is, what kind of information you’re getting. Right. So we believe that just knowing what diagnoses exist, what drugs these members might be on and the comorbidities is not enough. It’s great. It’s a huge leap forward to where we were just a few years ago. But from our perspective, what’s really important is the details.
So did this member go to the hospital in February, then came out of the hospital, got on a disease modifying therapy, only took it for two months instead of taking it for the full nine months that they were supposed to, stopped taking it, went back to the hospital, went to a specialist, then got on this step therapy medication after that.
Like that shows a pattern in the behavior of the person that is very predictive of what might happen next in that policy here. That policy that you’re underwriting. And so that’s those details are extremely important to us.
Ian Pietz
Yeah I love the detail there. If you’ve got access to the underlying claims data or some way to get access to the claims data, I think that’s part of the solution is finding ways to get access to the information. If it’s through third party vendors, things like that is one of the ways that we’ve seen these models being used effectively in the stop loss space.
And to your point on, not everyone has access to full data. I think some of the lines are blurring between the players: TPAs who are taking a risk and underwriting stop loss carriers who are doing underwriting, and better sharing of data. So while de-identified data or third party data is one path to get access to information, we’re finding that more and more players do have alternative paths to getting that underlying claims data. They can use different types of models that are built on those types of data sets.
Jason Oswald
Yeah. And I think there is some things happening in the market that it is difficult to get the data. It has traditionally been difficult, but the market is kind of changing a little bit. A lot of the MGUs or stop loss carriers that we talk to are talking about how big their fully insured takeover business was.
I remember probably, I don’t know, 12, 13 years ago, I would talk with brokers who were wondering if they can even get a quote on a two-hundred life group. You know, that would be their first move into stop loss. But now it’s just very common. Aggregating specifics, no new lasers, right? Those kind of tools that are kind of common in the market now really change what data is required and what teams will use to do their underwriting.
So it’s actually kind of allowed an opportunity to move forward with either different amounts of data than are traditionally available.
Mehb Khoja
Yeah. You know, let’s talk about data a little bit more. And I certainly don’t want you guys giving away any secret sauce. But the traditional approach for a stop loss carrier or MGU, everybody uses like an age/gender demographic, manual rate build up first, right? It’s, you know, the age of the individual, where they reside, what network they’re on, things like that.
And then to that, they mix the actual experience of the group so you can develop an experience rate. You can develop a manual rate. You blend the two and that’s basically the starting point of a stop loss rate. What data points do you guys have access to that are a supplement to what stop loss carriers are already doing?
Jason Oswald
Yeah, I think, so alongside of that, you’re talking again in traditional stop loss would be like a high claims report or maybe a 50/50 report or something. And some way to start adjusting the actual group experience with known claimants or something ongoing. What we’ve been able to bring there, and actually, I think what we’re seeing kind of broadly, not just in Gradient’s tools, but broadly is just more reliable access to the fully insured data and some of that information at a member level, even if they’re not high claimants, knowing somebody who’s just below that 50% level obviously provides a ton of value, especially in these AI type models that we’re using these days.
So I think it is the availability of it, I think Ian said it earlier about the availability of a lot of that data, even from a fully insured context and leveraging that in the stop loss space.
Ian Pietz
Not going too far down the rabbit hole in terms of what types of data, but different types of data give different, there’s different things you can do with it. Whether you’re talking about pharmacy data, there’s interesting things you can model off of that. Then broadly, the market started getting access to medical claims data as well. Now you can not only know what drugs are being taken, but how did that happen, where do those happen? why are they taking drugs? Is it a prophylactic? is it preventative? or is it associated with something specific? And you start to layer in labs data and all these other types of data. There’s other things that you can solve for, gaps you can find in the problem.
And to me it’s really about the trade off in terms of what you can gain out of predictive value compared to the cost of acquiring some of that type of informational level of coverage, because not all data sets are created equal.
Ali Panjwani
For us, it’s extremely important to have that transaction level detail to actually have the first dollar pharmacy and medical claims data on all members, or at least the majority of the members. And we realize that most of the carriers that we work with don’t have access to that level of information and so it was really important for us that we build the capability in-house to be able to take a census file, an enrollment file and acquire that first dollar of pharmacy and medical claims data on our carrier’s behalf, because the details are just so important to be able to predict what’s going to happen next accurately.
You know, two members are so dissimilar when you look at, you know, some of some of those details. So, we had to build in that capability before we came to market.
Mehb Khoja
Okay. So, you know, I go back to these tools being really useful in the fully insured space. They’ve now made its way into the stop loss space. But even with stop loss, you have carriers that focus on smaller deductibles. You know, think about like the captives and the level funded solutions. And then, you have carriers that focus on higher deductible, say like plus $250,000.
Is there a difference, do you guys feel like there’s a difference in the usability of your tools? If you’re talking small deductibles versus large deductibles?
Jason Oswald
I’ve thought about this, that I don’t know if there’s a difference in usability per se. I think there’s a difference maybe of trust. So in spaces with those higher deductibles that they’re already getting a lot of data, they’re very comfortable with the type of reporting that those teams are already receiving or they require.
And I think that there’s kind of a reluctance necessarily to change in those cases, strong reliance on internal manuals that are built with lots of good data over a very long amount of time. So I think that it’s not necessarily less usable in a sense, but they’ve already got good data. They have good processes. I think what I encounter is, if it ain’t broke …
Mehb Khoja
Resistance to change.
Jason Oswald
Yeah, resistance to change at those larger levels. Now, at the smaller deductibles with the smaller groups like there is an opportunity there. Right. And there’s a lack of data. And so I see, we see, a lot greater desire to move into these tools, to accept the tools on those lower deductibles, lower, smaller group sizes, just because there’s simply an opportunity to take on a lot of risk there, a lot of good risk and ensure these groups save them some money, make a profitable business.
So I don’t know if it should be that way, but it definitely is. Seems to be a reluctance, for the larger groups and an acceptance of the smaller groups.
Mehb Khoja
Sure. What do you guys think?
Ian Pietz
So when I think about low versus high deductible, I think there’s some scaling that happens there. When we look at the predictive problems associated with predicting incidence or the cost associated with 30,000 versus 150 versus 350,000, it starts getting very sparse in terms of the incidence of those high excess levels.
Mehb Khoja
Yeah.
Ian Pietz
I think that’s part of why fully Insured was quick to adopt as well, because you have a lot more data when you’re talking about an event out of a $700 PMPM that’s really different than $50 PMPM.
Mehb Khoja
Yes.
Ian Pietz
And so I guess what I’ve seen is that the predictive models are better at doing those lower thresholds. They’re more accurate, they’re more consistent. I think there’s a lot of value in those upper thresholds. But to Jason’s point, there’s also different levels of detail, and a different acceptance of risk. And carriers are more risk takers, they may be more willing to spend the manual time when you’re talking about a 5,000 or 10,000 life group, rather than trying to churn out 100 RFPs for 50 lives, for example.
So I think the value is there across the range of spectrum. But the predictive models have more work to do when you’re talking about very rare or accident related events that are often associated with those truly top end specs.
Mehb Khoja
Sure. What do you think, Ali?
Ali Panjwani
Yeah, I think both answers are great. You know, I look at it in two ways, and I feel like Jason and Ian addressed both, which is the financial feasibility of applying predictive analytics tools to groups with higher deductibles, generally larger groups. That’s one aspect of it. The other aspect is these tools’ ability to actually predict that risk more accurately than more traditional methods.
I think those are the two aspects that, that should be considered. Taking that second point first, what we have seen is that a model that works really well at predicting risk and claims spend in a lower deductible, smaller group environment is not the same model that works really well with predicting lightning strikes, you know, and just leaning on a couple of technical terms that I feel like, you guys will know.
Mehb Khoja
Lightning strike is a very technical term.
Ali Panjwani
Not that one, but precision recall, right? There’s a balance between you have to strike true positives and true negatives in a lower deductible model differently than you would do in a higher deductible model. And so I think a mixture of models from an accuracy perspective and those two scenarios is necessary. So that’s kind of the technical side.
The financial feasibility side, I think, we have some work to do as an industry. So I think today the use case is built very well for small groups. The amount of premiums that carriers can collect from a small group, with lower deductibles justifies the use of predictive analytics and, kind of, the pricing models that exist today. We have to do a little bit of work in making these types of tools financially feasible in a large group, high deductible setting.
Mehb Khoja
So, I’m also in the lightning strike business. Right. So we’re a reinsurer and these are really high dollar claims, right? Those that exceed $2 million. I’m specifically, really focused on gene therapy these days. Give me some comfort that your tools can help find claims that can exceed $2 million.
Ian Pietz
I think that’s an, I’m going to dodge that question just a little bit, but I think the gene therapy question is an interesting one because when you start talking about that type of spend, I think there is a question to be asked of, can you underwrite for that? And there’s really only decline or quote when you’re talking about identifying those types of risks, because there’s no level of premium that you could collect and spread across a book of business at a case level basis, I wouldn’t anticipate.
So to me, something that makes sense to move out of the underwriting process and look at a more of a top level payment type of a scenario. To the point of higher and more rare, it’s harder to predict, you know, I really haven’t seen predictive models that can do things at that level. I’m not sure that the technology is there yet.
Mehb Khoja
I feels like at that level, it’s just really about pooling rates, having enough lives to spread the risk across and really focus on pooling.
Jason Oswald
One thing I think we have going in our favor on this point, and I agree that the rarity of these therapies and how they’re being applied, makes it really hard to predict.
I think the one thing that we have going for us is that we know what conditions can be treated with this, and we can have some with these a lot of these cell and gene therapies, we know what information, who could possibly be a candidate for this and the ubiquity and usefulness of the data broadly that we can we can look at, can help find or at least exclude members to say, hey, they may have the underlying condition, but they are not a candidate for such and such a therapy.
So I do think there’s an opportunity to at least somehow exclude people who would otherwise be rejected just because of the availability of the data. Hard to predict overall. Maybe like emergent therapies, but if the underlying condition exists and we have some information that could help us say this is an insurable person who’s not going to take one of these therapies, I think that’s helpful.
Mehb Khoja
Here’s where I’ll say you guys have the advantage. Typically, when you’re doing the work you do, you try to collect the member level census, right?
So employers have employees. Employees have children. A lot of children right now are the ones that are getting gene therapies. And so you may not see it at the employee level, but because you guys are asking for member level census, you might be able to run against the data that you have that identifies somebody has spinal muscular atrophy or sickle cell. That might be information that the traditional reinsurer does not have access to.
Ian Pietz
And I think that’s fair. I think I think there’s a question of do you want to underwrite for that though, or do you want to pool for it? Because if I’m declining these very rare diseases, very rare treatments, am I declining so much of the population that I just don’t feel like I’m going to be able to run the business that way?
Mehb Khoja
Yeah, that is a core problem, right? Like right now there’s not enough premium to be able to absorb those types of claims. And so, by and large, the industry is leaning toward excluding those individuals which, you know, Ali to one of your earlier points, that doesn’t help the affordability and access to care. But stop loss carriers and reinsurers are also running a business, and they’re trying to protect their P&L. And so they can’t typically take on those types of claimants without some sort of pooling.
Ali Panjwani
Yeah, I agree, I just want to make a quick point. We’ve just had some really practical ideas on how to manage that risk. Right. And pooling is one, carve outs are another, I think that Ian mentioned. I have just a counterpoint to Jason’s point, around effectively eligibility of members in a population that might take gene therapy.
So this is a perspective that comes from my background in the specialty pharma space, in commercializing these really high cost medicines over the years, which is there’s a big difference between a member that is eligible to take a drug and one that is likely to take one.
So in that nuance, there are payers in the middle that make it really difficult. There are step therapies involved. There is patient out-of-pocket costs that sometimes make it prohibitively expensive. Sometimes these gene therapies require administration out of a center of excellence that is an hour or more drive away for a quarterly intrathecal application. There’s a lot of barriers to be able to take this. And so the main point that I want to make is, yes, it’s hard to predict.
I’ve seen tools try to do it. And I’ve seen tools overestimate the risk of it. Because we’re leaning towards …
Mehb Khoja
Conservatism.
Ali Panjwani
Yeah. Like eligibility. You know, how many members are eligible to take this medicine versus how many members are actually likely to take it after all those barriers are taken into account?
Jason Oswald
Sure, sure. Yeah. And that I think that was my point exactly that, like, if we could somehow whittle the pool to say, here’s ones who are more likely to actually take this, we can actually have populations get the stop loss coverage, right, who might otherwise be declined. So I think that’s a good point.
Mehb Khoja
So what does the future hold for tools like the ones that you know from the companies that you guys work for? What can we expect in the coming year?
Ian Pietz
I think, in the stop loss space in particular, more customization models and solutions that are that are well tailored to the business needs of individual carriers. I think we’re seeing that with some of the players in the spaces that they’re trying to find ways to differentiate themselves in the way that they write their business. So finding ways to meet that in the same way that they write their business.
Jason Oswald
Yeah. I hope that we’re going to see something maybe more consultative in the sense of, you know, saying this is a good spec level for this size of group or this risk of the group. So rather than saying, you know, kind of broad brushing, general risk statements at various levels, but actually helping to find the right levels, the right, you know, if an agg spec is appropriate, try to find something more consultative coming from the tools.
Ali Panjwani
The future, I think, contains products that better support larger groups, higher deductibles is one. And the second is better access to data. So solving the problem, Mehb, if I only have an employee level census, can we take it a step further as a partner to you and say, we will go ahead and access that member level census versus the employee level census and acquire the data and get you the insights that you need with full visibility of that group. I think that would be a game changer.
Mehb Khoja
I really like that answer. And this has been a fantastic discussion. So thank you, first of all, for engaging with me on it.
Let’s get you guys out of here with one more question. Make a bold prediction. You know, we’re in the stop loss and the reinsurance space. This is a $35 billion industry. And I’m curious from your perspective, what do you see happening this year?
Jason, why don’t we start with you?
Jason Oswald
Oh, man. Well, I know that there were, some, I don’t know how bold this is, I guess, you know, listeners can judge, but, I know that there were some challenges on the fully insured side. I think that we’re just going to see continued movement into the self-funded space by the fully insured market. And I think there’s going to be opportunities created there by the higher medical trends.
So fully insured transitions, level funded products, I think those are going to continue to grow.
Mehb Khoja
Ian, how about you?
Ian Pietz
To me, I think we’re going to see a lot more adoption of the types of solutions we’re talking about here. I think the market is primed, the players are here. There’s been competition in the space to raise the level of product being offered. And to me, this is a year when folks really take a serious look at things.
Mehb Khoja
And Ali, why don’t you close us up?
Ali Panjwani
Yeah. I’m going to make a prediction that is high level and self-serving, which is broader adoption of predictive analytics tools across all different types of carriers, small groups, large groups, low deductible, high deductible, I think that’s going to be good for the industry.
Mehb Khoja
Yeah. I would tend to agree with you. I think self-insurance will continue to grow. I think down market self-insurance has a lot of upside to it. And I think in that space you have to have access to different ways to underwrite. And so I would essentially agree with all three of you. And so let’s close it up there guys.
I really appreciate you joining for this edition of Firm and Final. And we’ll catch you guys next time. Thank you.
About the Podcast
Firm & Final: The Legends of Stop Loss and Reinsurance is an award-winning stop loss industry podcast from BCS Financial Head of Large Claim Solutions Mehb Khoja. With a new focus each season, Mehb brings together members of the stop loss, reinsurance, and self-funded industries to discuss current and future stop loss issues and trends, and share legendary experience and advice for the next generation of stop loss and reinsurance superheroes.
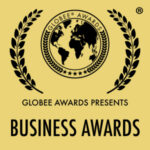
Record and submit your question at [email protected] to be featured on a future episode!
Podcast hosted by Mehb Khoja: linkedin.com/in/mehbkhoja
Sign Up To Get Updates From Us
"*" indicates required fields